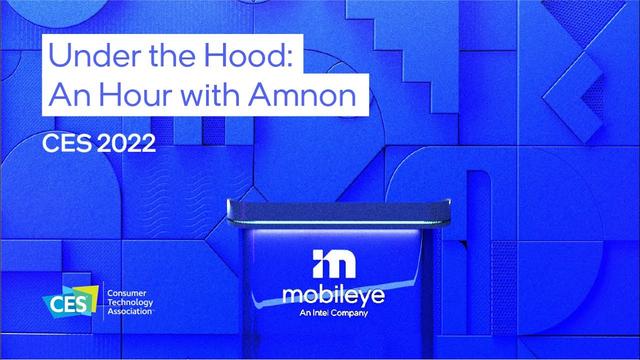
By cuterose
Intel’s MobilEye Levels Up To Take On Tesla And Others In Self-Driving
MobilEye, an IntelINTCcompany, announced at CES the newest generation of their custom chip for automotive driving, known as the EyeQ Ultra, a 176 TOPS processor with various specialized components they claim will be all you need for self-driving and a Robotaxi. Indeed, they plan to operate their own robotaxi services as well as partner with many other players wishing to enter that space. I sat down with Amnon Shashua, CEO and founder of MobilEye, to discuss their strategy. After reading this article, you can watch my interview to hear Shashua in his own words:
MobilEye’s efforts are serious, based on their long history in ADAS (their chips power the advanced driver assist and “pilot” systems in the majority of OEMs, with over 100 million chips shipped) and the ability to use that fleet of cars to gather data for training and mapping. As part of Intel, they have top-tier ability to produce custom processors. They are also using Intel’s silicon photonics and other resources to generate a new high performance LIDAR and imaging radar. They combine this with several unusual approaches and a system of safety constraints on their motion planner in hope of leading the field.
MobilEye’s efforts sit in constrast with TeslaTSLA, which certainly gets the most attention among car OEMs for their efforts, and Waymo, which is the overall robotaxi leader, though it has many competitors.
Shashua goes into a lot of depth on the strategy in this recent video. As it’s an hour long it’s more than most casual readers will watch, but the seriously curious should consider investing the time. There is also an edited 9 minute version, which you should view if you don’t have time for the full hour. I will summarize key points below.
MORE FROMFORBES ADVISORBest Travel Insurance Companies
ByAmy DaniseEditorBest Covid-19 Travel Insurance Plans
ByAmy DaniseEditorHere are the prongs of MobilEye’s strategy and key advantages:
This is a very impressive list, and I wrote about many elements of it a year ago. MobilEye continues to be one of the few companies in the space to do something surprising. In particular, that they have gotten places with the strategy of “ADAS with a better MTBF” is at odds with the philosophy of almost all self-driving teams except Tesla.
MobilEye vs. Tesla
It is interesting to contrast them with Tesla. Tesla is the maverick of car OEMs, capable of and willing to try things no established OEM will do. MobilEye used to be the company that provided the technology for Tesla’s early Autopilot, but they pulled out when an Autopilot accident killed the driver, and because they knew Tesla wanted to build their own system. Both Tesla and MobilEye have tried the approach of building up and evolving ADAS, while most other teams feel that self-driving is so different as to require a dedicated effort. Former Tesla Autopilot leader Sterling Anderson, who co-founded Aurora, called it “trying to build a ladder to the moon.”
MobilEye is famous for having built ADAS with a camera (and optional radar) where previously it was an expensive radar. They are camera-centric, but believe LIDAR and radar provide important, though secondary functions. More than that, MobilEye is actually building its own custom high performance LIDAR and radar. Tesla calls LIDAR a “crutch” that distracts you from the real goal of an all computer-vision system. It has recently been almost as mean to radar, and removed radar from future vehicles, though probably mostly because of the chip shortage.
One of Tesla’s biggest assets is their fleet, which gathers data to help them train their machine learning. There are well over a million Teslas out there, which take regular software updates and help in the quest. They also have a vast number of users for Autopilot who return data all the time, and a growing number of testers of the ill-named “full self driving” prototype they are building. MobilEye has a larger fleet, with 100 million chips sold, and they just did deals with more car OEMs which will result in 50 million more cars using their latest chips. Unlike Tesla, they can’t constantly update the software in the cars, nor get them to report the volumes of data Tesla can ask because the carmaker customers pay for the mobile data. But for both, this fleet is a big asset.
MobilEye goes further than Tesla and exploits the fleet for mapping, while Tesla disdains the use of mapping beyond the navigation level. MobilEye’s REM project creates fairly sparse maps, but includes more than just lane geometry. In particular REM watches cars as they pause at intersections, creep forward and make turns to know where the sightlines are, and just where the drivers actually drive — not just where the lines on the road are.
Both companies design their own custom chips to provide the processing power, since neural networks and computer vision are hungry for that. As part of Intel, MobilEye has a strong advantage here — it’s arguably the top processor company in the world. Tesla uses external chip IP and contracts with external fabs to make their chips, though they do a good job for a non-chip company.
There is also a difference in management style. Elon Musk is perhaps the greatest entrepreneur in history, but his style is brash, with no fear of hype or outrageous efforts and statements. Amnon Shashua makes bold claims, but doesn’t go nearly as over the top as Musk.
MobilEye “True redundancy”
MobilEye wants to claim a trademark on this, but it’s perhaps the most questionable element of their strategy. They are building two completely different perception stacks, one vision only, and the other using the LIDAR and radar. While in earlier statements they indicated both could drive the car, there is only one planner.
The basic philosophy that different systems will make different mistakes is a strong one, but only to a point. The errors the two systems will make are not entirely indpendent. If your vision system fails once in 10,000 miles and and your LIDAR/RADAR fails at the same rate, you definitely not going to get a system that fails every 100 million miles — not even close. The MobilEye approach was described by Shashua as “an OR gate” meaning that if either system detects an obstacle, then one is viewed as present. This reduces your false negatives (blindness that can make you hit things) which is good, but also increases your false positives (ghosts you brake for.) Generally false positives and negatives are a trade-off. You can’t have blindness, but if your vehicle constantly reacts to ghosts it’s not a usable system.
I liked a @YouTube video from @kerondisney https://t.co/AvZICb5XAf HOW TO REMOVE SCOOTER WHEELS AND REPLACE THEM
— razzer Wed Oct 19 18:40:25 +0000 2016
Shashua realizes you don’t get to multiply the MTBFs but feels he will still get something “much, much better.” Most other teams try something more complex in their sensor fusion, rather than an “OR.” They try to fuse returns at different levels, starting at basic sensing, but sometimes going all the way to after classification. It’s not clear why the MobilEye approach is superior, except from a software engineering standpoint, as you can just put two different teams on the problems and not worry too much about integrating their work. He asks how the system will perform if you “shut down all the cameras” or the LIDARs. He states that you can look at his systems being tested in many cities and they are performing at a much higher level than purely camera based systems. For camera based system he asks, “If they shut down a subset of their sensors could they continue? ... I think not.”
MobilEye is also creating a “VIDAR” — a virtual LIDAR that attempts to make LIDAR like point clouds from 2D camera images using machine learning. Many, including Tesla are working on this, and it shows promising results but is not yet at “bet your life” reliability. That’s one reason they also have the LIDAR.
Indeed, the new imaging radar and LIDAR look impressive, though only modest details are revealed. They even have an experiment to see what it looks like if they take the imaging radar and try to turn it into an image video using deep learning — a challenge when you consider how little resolution is in even the best radar. Radar’s ability to see through most weather is a big plus in places where that’s crucial. Radar’s other big edge — knowing the speed of all returns thanks to Doppler — is also found in FMCW LIDAR. Indeed, if you have FMCW LIDAR, the virtues of radar are fewer. In addition to the weather penetration, it is cheaper (MobilEye plans only one forward facing LIDAR) and it also can see “invisible” objects because radar waves can bounce under cars to detect a vehicle 2 ahead of you that’s hidden by a big truck, at least if it’s moving.
Shashua is optimistic about his imaging radar. It can “with the right development become a standalone sensor. Today radar is not a standalone sensor.” In the future “It can compete with a LIDAR and then instead of having 360 degree LIDAR, you have only a front facing LIDAR and you can bring the cost down considerably.”
MobilEye REM maps
In keeping with MobilEye’s quest for what might be described as the “Goldilocks” point, their mapping system does not have the high detail of those from Waymo and other companies, but it has much more information than a “no HD maps” player like Tesla. REM maps, MobilEye states, take only about 10 kilobytes per mile, a cost which fits in the budget of the mobile data plans in the cars of their customers.
In the REM system, cars with the chips are using them to locate important road elements, including objects in 3-space, signs, lane boundaries, traffic signals and more. They are compressed down and uploaded if changed. In addition, the cars report their driving tracks (which can be accurately placed on the map.) These tracks reveal not just what is painted on the road, but what large numbers of cars have actually driven. Natural human driving often involves not being centered in the lane or taking an exit as drawn. MobilEye has noticed the common problem of unprotected turns, where cars must creep forward until the driver (or cameras) can see what they need to turn. Using the REM data, cars can know just where they need to get in order to see what they need to see, resulting in a more human-like driving pattern with less uncertainty. This also collects what might be called the unwritten rules of the road, the rules that human intelligence figures out, and makes them part of the map.
With the largest fleet, MobilEye equipped cars are likely to encounter any changes to the road quickly. This is not just the robotic fleet, but all the human driven cars able to handle construction zones and other changes, and even teach how to drive in them. The risk of coming upon areas where the world has changed from the map is overstated — all cars must be able to handle a wrong map gracefully, and for each construction zone or other change there is only one car that is the first to encounter it. MobilEye has the advantage that this is often a human driven car, making it unlikely any early robotaxi will be the very first, forcing it to exercise its “drive with a wrong map” skills. That’s in contrast with Tesla where the car has to use its “drive with no map” skills all the time.
RSS planning system
Driving safely is one (though far from the only) important factor in making a working self-driving car. The challenge is to be safe while also being a good “road citizen” which includes some aggressive behavior in order to make traffic flow in a large number of cities, especially MobilEye’s home territory of Israel. Chaotic driving there has led them to develop a set of rules for planning the car’s path that they call RSS (Responsibility sensitive safety) which constrain and enable paths for the car, keeping it’s actions legal and reasonably safe. Though it could be argued the approach guarantees the vehicle won’t violate the vehicle code, though that might involve it in unsafe situations because other vehicles ignore the code. Regular driving involves such situations regularly, and MobilEye is one of the few to talk about solving them.
That said, access to data about MobilEye’s real world performance is currently modest compared to what we know about some other companies. They are pushing for RSS to become an international standard, to get regulators to demand that RSS be implemented to get certified. I suspect more real world testing (or at least reporting) is called for before this is done.
Robotaxi plans
MobilEye is planning both to sell hardware and systems to carmakers, and also to build and deploy its own Robotaxis. MobilEye purchased MoovIt, a multimodal trip planning app, and is using it to allow users to book trips in its robotaxi pilots. It has stated it will begin robotaxi pilots in several cities this year and in the coming years. At the same time, it is helping Geely’s Zeekr produce its own Robotaxi with multiple EyeQ5 chips, and supplying delivery robot company Udelv with systems to drive their unmanned vehicles, with deployment not yet announced.
Shashua expects a world of “co-opetition” where suppliers are competing with their own partners. Certainly many of MobilEye’s customers plan their own robotaxi operations, either with MobilEye chips, or in cases like Ford, through the different system made by Argo.AI. This willingness to both supply car OEMs and startups and also operate its own service seems brash, but it positions the company as one of the few companies with efforts in both consumer cars and robotaxis, not worrying too much about which will win. (Or, in fact benefiting from the reality, which is that neither will overwhelmingly win for a long time.) Tesla plans to play in both areas in a clever way, but unfortunately with inferior hardware that relies on a longshot approach.
One thing still missing from the MobilEye story is real data about its robotaxi efforts. There are scores of teams developing robotaxis, all making big claims. Only a few, though, are backing up their claims by letting the public see an unvarnished picture of their performance, with real statistics, and allowing unvetted and unscheduled rides by members of the public who can publish videos. MobilEye has released nice videos of their vehicles driving various routes, as have many firms. These videos show sufficient capabilities to demonstrate that MobilEye is a player, but it’s a very, very, very, very long journey from that to having a working service.
Many of the signs from MobilEye are good, and the collection of strategic moves is superb. The proof, though, is in the quality of their system in a real robotaxi environment which we must wait to see. In the 2010s it was sufficient to show plans and research. Today actual operations and commitments are what matters, as outlined in the milestones of a robotaxi service. When a company actually does things, like deploy unmanned vehicles, it proves to us that their board of directors signed off on taking that big risk, which in turn means that their internal research said they were ready to make a “bet the company” move. For now, we only have MobilEye’s declarations that their “evolved ADAS” approach has surprised us and done the jobs, and we need to see those declarations made real. They probably won’t hit their target of “early in 2022” but promise that thanks to REM and other tools, they can deploy quickly in new cities with minimal effort.
At present, people have not been paying as much attention to MobilEye’s efforts nor valuing them the way that some companies have with dekaunicorn status. MobilEye used to be a public company until it was bought by Intel. Inside Intel, its efforts have not been able to move the needle of the chip giant’s valuation. This may be why Intel plans to spin-off MobilEye in a new IPO shortly, which Shashua could not comment on. It will be an interesting stock to watch.
Are we done yet?
Shashua believes that the robotaxi problem is close to solved. So close, in fact that he doesn’t think we’ll need more algorithmic breakthroughs, and as such we can say today what hardware is enough to do the job — and that’s the hardware he has put in the EyeQ Ultra chip. Indeed, they feel that 6 to 8 of the EyeQ 5 chips they offer today can do the job, which is what gives him the confidence that the EQU is enough.
That’s a fairly bold claim, because the history of the research teams that are the industry has been one of finding new techniques, and that has informed what hardware we actually want. But if you are a chipmaker, you have to decide what goes in your chip so you can tape it out and get it into production 3 years from now, so you need to choose well. MobilEye got lucky early on. They designed their earliest chips before neural networks exploded on the scene, but those chips had GPU-like elements for massive parallel processing that were able to run earlier, smaller neural networks. Now it’s not luck (and they might not call it that, but frankly very few could have predicted the big deep learning explosion of the early 2010s) and they have made their plan.
Cost
Most robotaxi developers aren’t strongly focused on cost. Almost all started using very expensive LIDARs that clearly cost too much for a production vehicle. They made the correct bet that the cost of the extra gear would drop greatly by the time things were ready to deploy. When your only goal is to get to market first by being safe first, cost is not that much of an issue.
MobilEye came to this by a different path. They began by making a camera based ADAS tool that could do things like adaptive cruise control for less than the automotive radars of the day. In addition, they could do it better, like handling stopped vehicles. They did very well with this. As they have tackled self-driving, cost has remained an issue for them.
The Ultra is planned to cost less than $1,000 in volume by 2025. The LIDAR will have an MSRP of about $1,000. While other vendors promise $250 LIDARs and Shashua says they could also produce on at that price, theirs will be higher performance and worth that cost. The full package of chip and sensors will come in “way below $5,000.” That cost of parts typically adds $10K to $15K to the cost of a consumer vehicle, but is a pretty reasonable cost addition to the cost of a robotaxi. Indeed, Larry Burns, a former VP of GM who consults in the industry, estimates that all the things you remove from a robotaxi — wheels, pedals, most of the dashboard, adjustable seats, mirrors and more — can easily cost more than the cost of the new additional sensors, making the robotaxi cheaper than a similar sized car.
Conclusion
MobilEye is one of the few companies to have it all: Experience, a huge fleet to draw mapping and training data from, extensive mapping efforts at very low cost, an FMCW LIDAR in the works, imaging radar, advanced computer vision, a trip-planning app, the ability to make its own silicon, low cost, robtaxis driving in complex cities and the most relationships of automakers of anybody in the game. While some players have better in some of these individual area, nobody has as good a combination. The key that remains to be seen is just how good their software is. Shashua said they are still working at getting their system to 1,000 hours between accidents but they are confident they will get there soon. That’s not there yet, as humans go 3,500 hours between minor dings and about 12,000 hours between police reported accidents. We’ll be watching to see how they do.
Read/leave comments here.